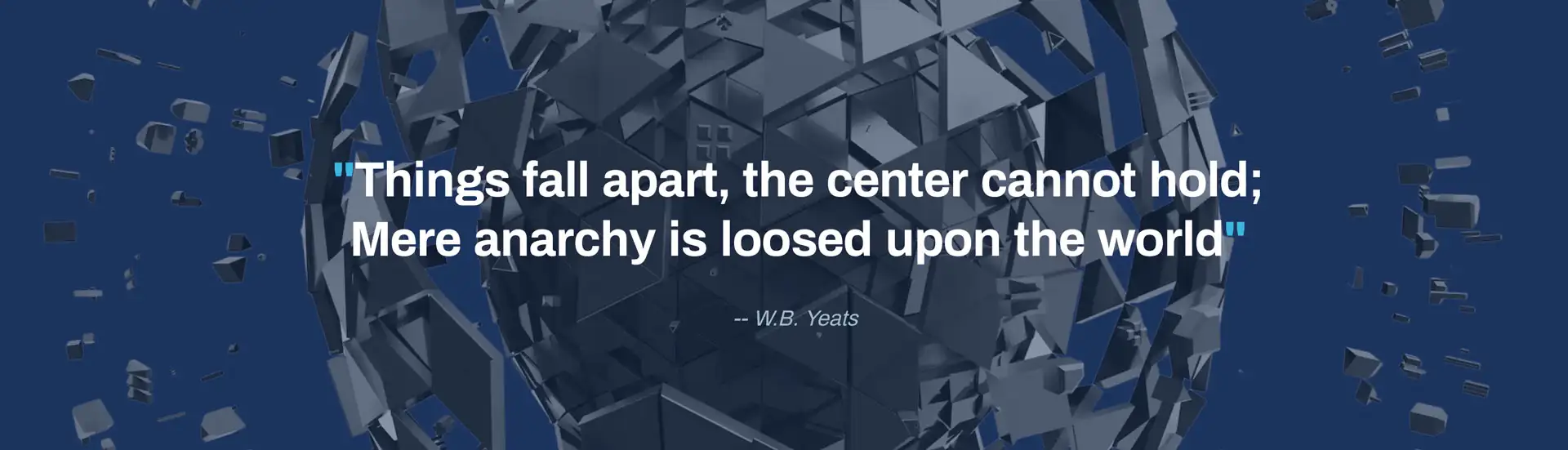
The industry knows the problems, they have been top of mind for years. Log volume is out of hand, log visibility and SIEM costs are at odds, skilled security analysts are hard to find, hard to train, and hard to retain. The security industry could be seen as being a bunch of pack rats, just trying to store as many things as possible. That was something to point at and try to trim and discard, however; industry compliance requirements and other regulations are demanding more and longer storage of logs and information.
This is all on top of the oldest problem in Security Operations: this effort is hard to do well.
To use an old example, the industry has tied itself in what could be styled a gordian knot, and in order to untie the knot the industry has provided several tools, processes, and systems that busy different edges of the knot and untie bits and pieces here and there.
Deepwatch doesn’t have the answer to all problems in the Security Operations space. No one person or organization does. That being said, how about a different approach to the knot? Instead of working from the edges in, step back and consider the problem in a more simplistic, Alexander the Great-like way.
We must address external and internal pressures, an avalanche of data and costs, and a lack of time to handle all of the events that are presented through the analysis of as much of that data collected as possible.
Addressing External Data Pressures
Omer Singer at AnvilLogic describes where he believes the industry is going on external data as:
“The cybersecurity ecosystem is reshaping itself. The technology, the leaders, everything now is shifting so that security teams can have a more open future – a future where they’re not locked into a single SIEM, one with freedom for detections, and freedom for response.
From data pipelines to threat detection platforms, an unbundling is taking place. Security organizations increasingly prioritize flexibility and optionality, driving demand for decoupled solutions. Analytics separate from data storage, stand schemas and open table formats are all gaining mindshare.”
Omer Singer AnvilLogic
Omer is right over the target, addressing the external pressures from data overload is going to be counter intuitive. Embracing more data, but having that data be more structured, readily available, and allowing the freedom to utilize several data analysis tools at the same time will increase visibility and reduce costs.
A decentralized security data plan allows for highly regulated or compliance data to reside in an accessible location, with a cost structure that minimizes search and maximizes storage costs. High fidelity security alerts, along with relevant data for enrichment, validation, triage, and response must be processed through a correlation engine to maximize search and correlation and minimize storage. This is the data freedom that Omer is talking about.
Multi Cloud and Cloud to Cloud scenarios also need to be taken into account. At Splunk.Conf last year, Splunk made the following announcement:
“Among the many announcements we are sharing at .conf23, one of the most exciting is Splunk’s new strategic partnership with Microsoft to build Splunk’s cloud solutions natively on Microsoft Azure. Together, our approach will enable our joining customers to migrate, modernize and grow their environments with end-to-end cloud and hybrid visibility at scale.”
Gary Steele Splunk
In short, Splunk is actively working with Microsoft to enable customers to move their entire Splunk analysis infrastructure between Splunk Cloud in AWS over to a different cloud provider. This is some of the flexibility that the industry is looking for, and is helpful as there are cloud-to-cloud costs that need to be taken into account. If a company is heavily invested in the Microsoft ecosystem, moving all of those logs, alerts, and events into a different cloud is not a trivial cost. It is certainly more cost effective, for the customer and the cloud provider, to keep that infrastructure in a single cloud.
This still doesn’t completely reduce the data burden. We need to be able to leave data in different locations or sources, multiple cloud providers, on-premise locations, as well as collect alert and event data from different security detection tools, while focusing our ingestion and correlation capabilities through a single best of breed SIEM, or looking at multiple SIEMs or independent data lakes that allow for a malleable detection surface while allowing the prioritization of alerts and threats to be presented to analysts through a single interface.
Addressing Internal Pressures
Solving for internal pressures is all about maximizing the amount of time available to security analysts to move through their alert queues, while minimizing the amount of time needed to take a response. It is incredibly important to recognize the ability to take the right action at the right time, so as to not only handle the present threat, but to also set in motion the protections, changes, or controls that will mitigate the next related threat from being an issue. This saves time for analysts and removes the drudgery of the same alert, with the same response over and over again. Call it having a “Case of the Mondays” in the SOC.
Time can also be saved as part of the investigation process by helping analysts with additional context or history: prior responses actions, asset or identity risks and current status, existing controls, all in conjunction with standard enrichment capabilities such as: related observables, sandboxing, authentications, scope, etc.
How does this save time? One word, Hyperautomation. Deepwatch is leading the charge to move beyond Managed Detection and Response into Cyber Resilient Security Operations through the use of Hyperautomation and our next advancement of an Open Security Data Architecture. Hyperautomation allows for more flexible automation and correlation of alerts from disparate or non-monolithic sources.
This also allows for data flexibility while providing the necessary aliasing or normalization of structured or unstructured data through multimodal genAI capabilities. Hyperautomation also powers the ability to reach back out to these flexible data sources, data lakes, or other SIEMs for enrichment and verification sources. It saves analysts time in the investigation, and to reach data where it is, not to force ingestion and normalization ahead of time into a monolithic data location.
The Deepwatch Open Security Data Architecture will provide hyperautomation this flexibility in advanced security actions, along with providing an internal data lake which will be powered by the multimodal genAI. This data lake will provide the reference-ability, history and context needed to provide the coordinated responses that move an organization’s security program forward toward becoming cyber resilient. This makes your organization more secure tomorrow than you are today, and gives that valuable time back to your analysts or security experts. It gives them more time in their day for other tasks, training, hunting, patching or other security improving actions.
We detail much more of these concepts in the Open Security Data Architecture around Multi-Cloud, GenAI and data implications, along with more on saving analysts time.
Share